A Brave New Intelligence
From proving mathematical theorems to appreciating sunsets, how far will AI go?
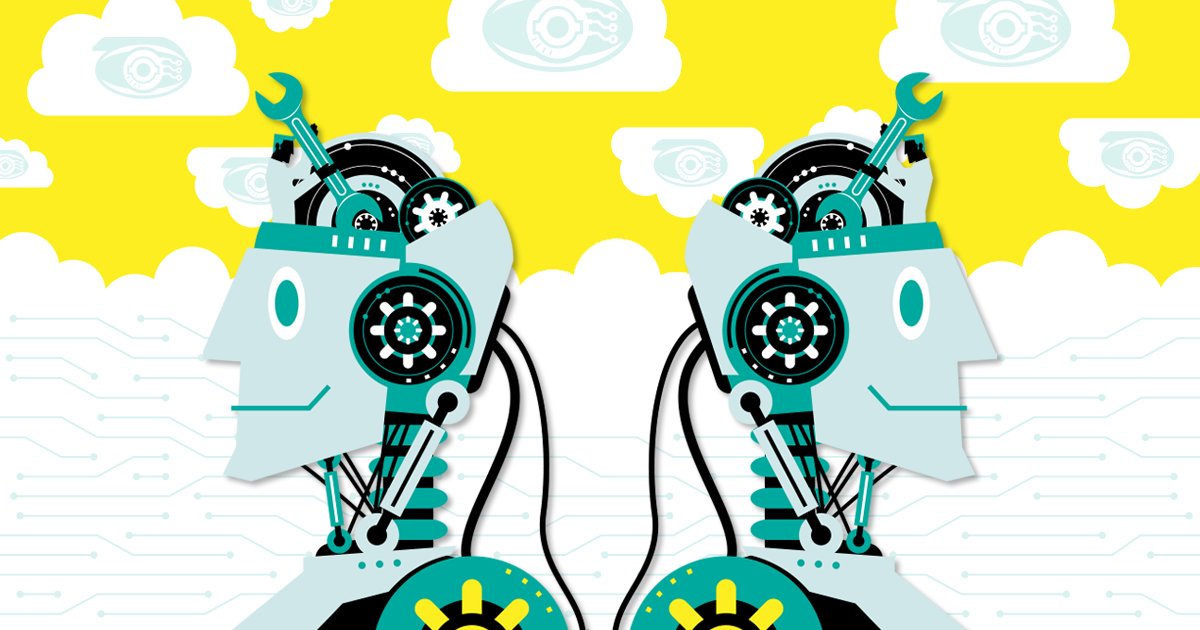
The sunset bathed Aidan’s sensors, stimulating the robot’s optic preprocessing before being transferred to a series of neural networks. Daubs of pink and orange faded into streaks of ever-graying violet against the darkening sky. Aidan drew in the wavelengths, letting them course through its myriad circuits, as it pondered the uniqueness of the moment.
A young woman, Romy, sat on the ground beside Aidan as they both gazed out at the horizon. “Penny for your thoughts,” she said. The phrase was an anachronism. Physical currency and coinage had been abolished nearly a century before.
“Mostly I’m letting my internal processes drift,” the AI replied metaphorically. “I find it’s healthy to experience my default mode on an occasional basis. But I suppose I was contemplating the natural beauty around us. This sky is like something out of a Turner painting.”
“I know what you mean,” Romy replied. “It’s overwhelming. The intensity, the grandeur. Sometimes when I see a sunset this amazing, it makes me so happy I want to cry.” The young woman considered her words a moment before adding, “How does it make you feel, Aidan?”
The AI pondered her question, thinking about how its processing differed from paradoxical human emotions. Finally, it answered. “It’s difficult to express. Let me get back to you on that.”
What is it like to be an artificial intelligence? Certainly, we can’t currently answer this question, given that at this stage of their development, two decades into the 21st century, no AI can experience anything so far as we know. But will this always be the case, and how will we know when the nature of technological intelligence changes?
It should go without saying that we are nowhere near being able to build an artificial intelligence of the level described in the opening scenario. So many challenges must be met before this could happen, and even then, there’s no guarantee AI would ever be able to appreciate a sunset or experience the world as we do. But if technological intelligence is ever to approach such sophistication, what are some of the hurdles that must be overcome and what will it take to achieve this?
Origin Story
It’s easy to forget that artificial intelligence is still a very young field. While a few earlier dates can be pointed to, most people would say the field and even the term artificial intelligence really got their start at a summer workshop held at Dartmouth College in 1956.
Attended by more than 20 researchers and academics, many of whom would become luminaries in their own right, the workshop had high expectations of what it could achieve in a mere two months. According to the project proposal authored by John McCarthy, Marvin Minsky, Nathaniel Rochester, and Claude Shannon, “an attempt will be made to find out how to make machines use language, form abstractions and concepts, solve kinds of problems now reserved for humans, and improve themselves. We think that significant advances can be made in one or more of these problems if a carefully selected group of scientists work on it together for a summer.”
Needless to say, this was an ambitious objective indeed. Still the group — both as individuals and working in concert — made headway in a number of these areas, including the development of “Logic Theorist,” the first true artificial intelligence program, which was designed to prove mathematical theorems.
This first era of AI focused on symbols and logic and so is referred to as “symbolic.” This was the predominant approach until the late 1980s. Sometimes called good old-fashioned artificial intelligence, or GOFAI, this handcrafted approach achieved many breakthroughs but also had numerous shortcomings, including its inability to learn in an unsupervised manner. Additionally, it suffered from brittleness, a term used to denote a program’s tendency to fail completely when conditions or variables fall outside a given range.
Artificial intelligence funding has seen a number of ups and downs over the decades with two significant pullbacks due to promises and expectations far exceeding the reality of what could actually be delivered. These periods became known as “AI winters,” and while the exact years are sometimes debated, the first is usually said to have lasted from 1974 to 1980 and the second from 1987 to 1993.
A second paradigm of AI that has become more prevalent in recent decades is known as connectionism, a reference to the many different types of connectionist neural networks it uses.
This approach was inspired in the 1940s and 1950s by the relatively recent discovery of the biological neural networks that make up mammalian brains. In fact, there was considerable dispute even then (as there still is today) over which was the more promising paradigm for eventually achieving human-level AI, the symbolic or the connectionist approach.
Each side believed its methodology was the key to building something comparable to human intelligence. However, in those early days it was almost a foregone conclusion because the necessary processing power for even the simplest neural networks simply didn’t exist yet. It would take several decades of exponential growth as forecast by Moore’s law to deliver the necessary computing power needed to make neural networks a viable approach that could move beyond the research lab.
Though work continued to be done on neural networks in the 1980s and 1990s, it wasn’t until the 2000s that they would really make their mark. Advances in both algorithms (sets of instructions) and processing power soon made possible capabilities once only dreamed of. Rapid image classification, superhuman performance at games such as Go and Shogi, natural language processing, language translation, and even world-class poker were among the many AI accomplishments of the second decade of the new millennium.
Also known as deep learning, this form of machine learning uses a series of successive layers of nodes (inspired by neurons) to extract different features from the input data. In the case of image detection, for instance, different layers might focus on edges, surfaces, and other features.
The Third Wave
Many significant advances could be in store for AI as we look ahead at this coming decade. Where the symbolic and connectionist paradigms have been described as the first and second waves of AI, respectively, work is now underway on a third wave that, if successful, will utterly transform the field and probably the world as well. It is said that where the first wave described and the second wave categorized, the third wave will explain. Focused on contextual reasoning, this approach would allow AI to one day understand context and meaning, something it really doesn’t do yet. This will be essential if AI is to continue to grow in its usefulness as a tool.
For instance, current neural networks can identify a dog and even determine what breed it is. Going beyond this though, third- wave AI would be able to explain what makes the image a dog, anticipate how the dog might behave in different circumstances, and explain how its algorithms reached those conclusions. This is all extremely important because AI doesn’t currently have a contextual understanding of images or anything else that passes through its circuits. It simply arrives at a probability showing the data it has processed correlates with a series of previous examples and labels it acquired during its training.
The other major issue this addresses is explainability. With the current state of the technology, AI processing is what is known as a “black box.” It arrives at its output, but neither it nor its human programmers are able to describe the logic or steps that yielded the result. This is unacceptable for so many applications where such understanding is essential, even critical. Whether controlling complex utilities, engaging in military chain-of-command decisions, or ensuring biases aren’t creeping into decision processes that affect people’s lives (such as whether or not to accept a loan application), explainability will allow us to trust these devices to a far greater degree than we can today.
Another feature being developed in this third wave is systems with common sense. Being able to identify that something is a car or a dog or a person is useful, but knowing how that object interacts with its environment will make AI a far more powerful tool. For example, if I drop a raw egg, I understand it will be smashed into a gooey mess when it hits the kitchen floor. If someone asks if an elephant will fit through my front door, I know the answer to that too. I also understand that I’ll burn myself if I hold my hand too close to a candle flame for an extended period. A computer doesn’t know any of these things, and this is a real problem as we seek to entrust AI with our increasingly complex systems.
Related to this capability is an understanding of causality. Human beings generally understand that if something happens, certain reactions are likely to result. If one person hits another, the struck person might cry, or hit back, or run away, or perhaps they’ll take some other action. We also know that the person being struck won’t suddenly turn into a balloon animal or disappear into thin air. Such an understanding of causality would make these systems incredibly useful.
If one person hits another, the struck person might cry, or hit back, or run away, or perhaps they’ll take some other action. We also know that the person being struck won’t suddenly turn into a balloon animal or disappear into thin air.
Another desired quality in this next generation of AI would be the ability to learn from very limited examples, methods commonly known in deep learning circles as one-shot learning and zero-shot learning. In the former, learning occurs based on very limited examples, incrementally building knowledge, much as people do. Currently, neural networks require enormous data sets for training. Thousands, even millions of examples might be used to statistically extract the common features that allow it to identify a cat as a cat and not a dog. With one-shot learning, a handful of images or even a single picture may be sufficient for a system that learns more like we do. In the case of zero-shot learning, identification is inferred from prior knowledge even though the observer has never seen the object or experienced the situation before. As an example, if you know a zebra looks like a horse except that it has black and white stripes, you would be able to identify the animal even without having previously seen one.
It has been theorized that this use of object classes is a critical part of how children learn over time. By some estimates, we are eventually able to recognize and discriminate somewhere between 5,000 to 30,000 different object categories. And this is but one of the many key building blocks of human intelligence. According to cognitive psychologist Elizabeth Spelke, there is a series of core domains of child cognition that include objects, agents, places, numbers, forms, and social beings. This acquired framework combined with our incremental understanding of causality and common sense allow us to navigate and interact with our environment as no other animal can.
There are many more approaches to improving machine intelligence that fall under this label of third- wave AI. Research institutes and programs all over the world have focused their attention on one or more of these. For instance, the Seattle-based Allen Institute for Artificial Intelligence, or AI2, is working on several of these areas including the field known as machine common sense (MCS). Led by University of Washington computer science professor Yejin Choi, AI2’s MCS program Mosaic is focused on creating “universal representations of common sense that can be shared and used by other AI systems.” To this purpose, one of the program’s web-accessible projects is Iconary, a modified version of the drawing game Pictionary. The primary difference is that Iconary has a human user play an AI in a game of collaborative communication. The two players then take turns drawing scenes from which a phrase or concept is guessed at by the other player. Over time, the AI becomes trained about different aspects of common-sense reasoning. By crowdsourcing the training in this way, the workload becomes much more manageable while also raising public awareness about the machine learning process.
Duke University, MIT Media Lab, Stanford University, and the University of Washington are among the many institutions working on different aspects of next-generation AI. At University of Massachusetts Amherst, Dr. Hava Siegelmann leads teams focused on the understanding of biologically inspired computational systems. One of these areas of focus is lifelong learning machines (L2M). L2M seeks to develop machines that incrementally build an understanding of the world and its concepts much like humans do. According to Siegelmann’s four pillars of lifelong learning machines — internal exploration, context-modulated computation, continual learning, and new behaviors based on the accumulated knowledge, L2M “will enable systems to continuously improve based on experience.”
Such cutting-edge capabilities would certainly offer considerable strategic and tactical advantages, so it should be no surprise that the U.S. military is very interested in this research as well. In September 2018, DARPA, the Defense Advanced Research Projects Agency, announced its $2 billion AI Next campaign with the goal of developing systems that “will function more as colleagues than as tools.” Focused on many of the hard problems of AI, the five-year program is targeting a range of promising research. Just as its previous support jump-started technologies such as self-driving vehicles, brain-computer interfaces, and even the internet itself, DARPA hopes to advance the field of AI in order to maintain an advantage on the global stage.
The Future of Intelligence
This third wave of AI is only one of the many ways our world is set to become more and differently intelligent. In the coming decades we’re likely to see the development of technologies that will radically change the ways we interact with our world and with each other — brain-computer interfaces that allow us to have near-instant access to information and processing resources, biotechnological augmentation of our natural cognition, digital clones that can act as our emissaries between the physical and virtual worlds. Most certainly these will be met with both acceptance and condemnation, with eagerness and apprehension. It will hardly be the first time new technologies have elicited such a tangle of reactions.
Of course, none of this would be possible in the first place without the unique capabilities of the human mind. Our creativity and curiosity are what will drive so much of this new era of technology. But whether any of this results in AIs that can actually think about and experience the world as we do, only time, and a great deal of work, will tell.